Human Knowledge Drained: AI Models Now Feeding on Their Own
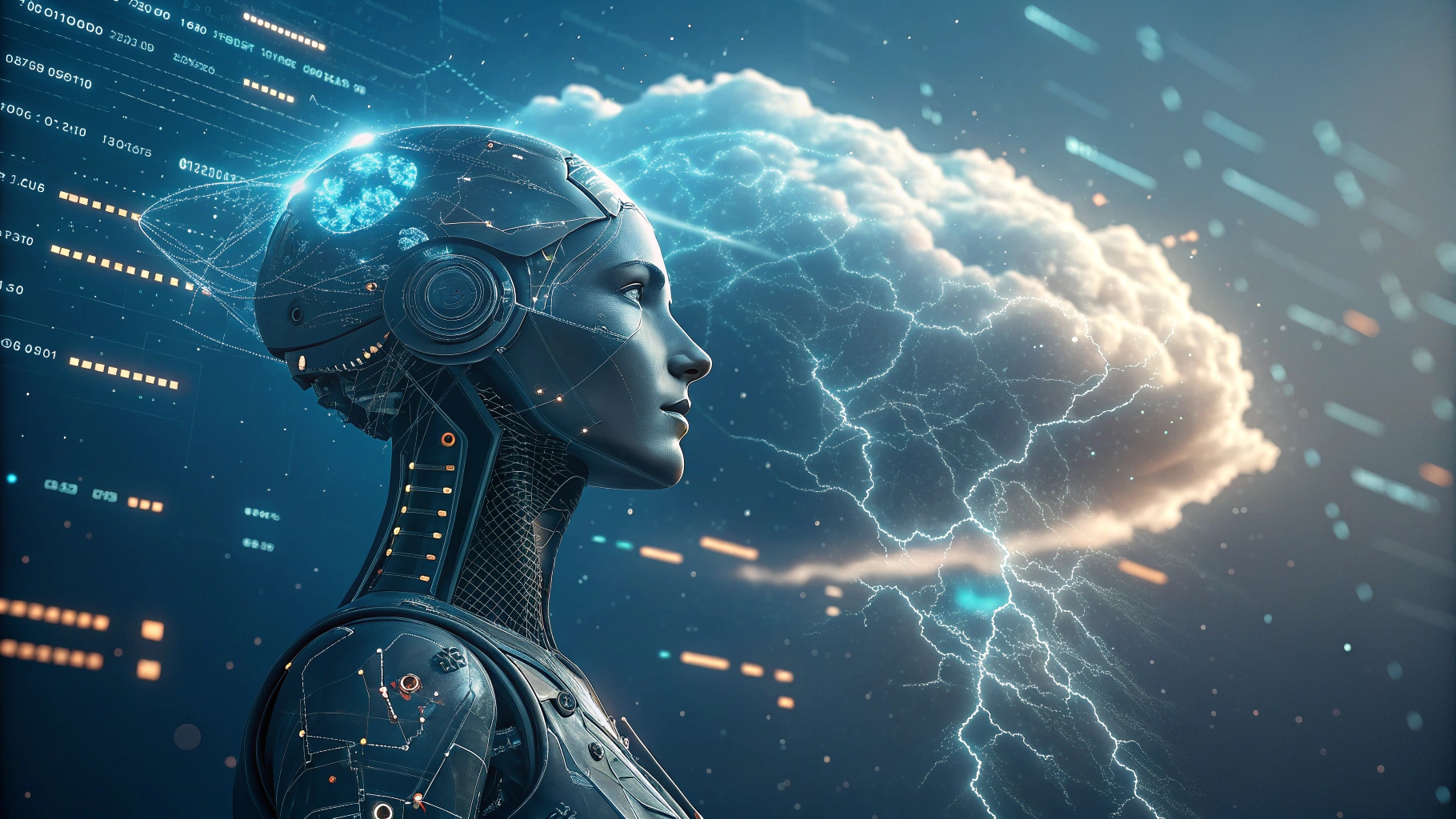
Table of Contents
Introduction
Have you ever paused to consider how artificial intelligence (AI) works? Every smart assistant, chatbot, or recommendation system relies on massive datasets of human-generated content to function. These AI models learn from billions of pages of text, capturing language patterns, contextual nuances, and even societal norms. But here’s the catch: by late 2024, the tech world hit a startling milestone—AI models had exhausted all high-quality human data available for training.
Now, AI developers are turning to synthetic data—essentially AI generating content for itself to learn from. It’s a fascinating yet concerning shift, and I’ve got a lot to share about its implications, both good and bad. Let’s dive into why this is happening, what it means for AI’s future, and how it could impact our lives.
The Dependency of AI Models on Human Data
AI models are like sponges—they absorb knowledge from everything we, as humans, produce. From online articles and academic research to social media posts and even comments sections, these models learn by analyzing enormous amounts of text. For instance, OpenAI’s GPT-4 was trained on over 570GB of textual data, equivalent to billions of pages of written content. That’s a staggering amount of information!
These models depend on diverse human input to understand context, tone, and logic. This dependency explains why they’ve become so adept at mimicking human conversations and performing complex tasks like summarizing articles or writing essays.
But here’s the thing: as AI capabilities have grown, so has the demand for even larger and richer datasets. According to a 2023 report, over 1.5 trillion words of publicly available, high-quality text had already been processed by major AI models. That’s almost all the accessible human knowledge on the internet! When you think about it, it’s like running out of gas on a long road trip—the engine needs fuel to keep going, and for AI models, that fuel is data.
Data Exhaustion: A Problem of Scale
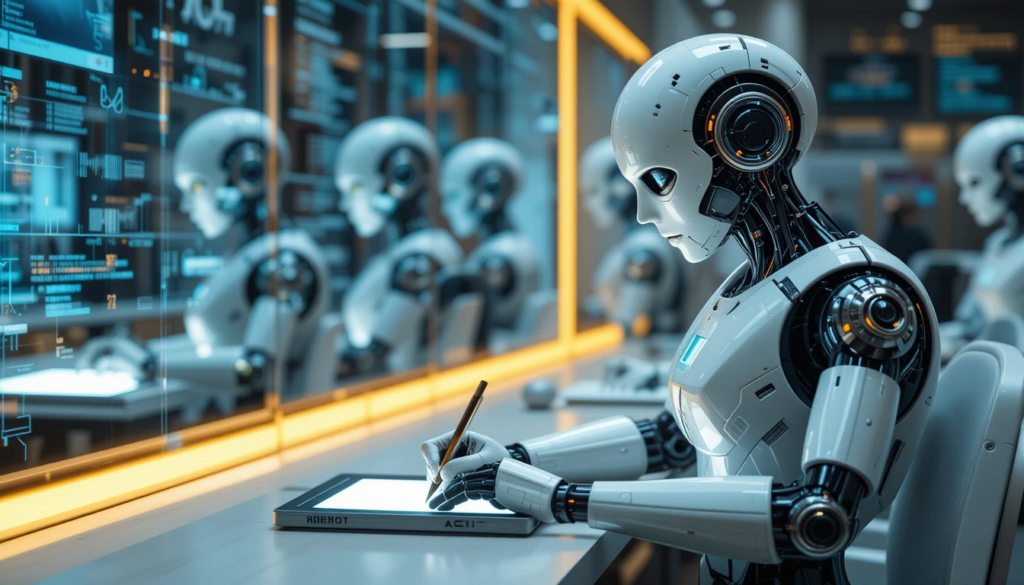
So, how did we get here? One reason is scale. AI research has grown exponentially, with more companies building sophisticated models for everything from language translation to drug discovery. As these models become more advanced, their data requirements balloon.
But there’s another layer to the problem: legal and ethical constraints. In 2023, OpenAI and other tech giants faced lawsuits for allegedly scraping copyrighted content to train their models. Many creators and publishers began demanding compensation for the use of their intellectual property, further limiting the pool of usable data. As someone who has blogged for years, I understand their frustration. Imagine pouring your heart into creating something, only to have it used without permission to train an AI model that could potentially outcompete you.
These limitations have forced companies to explore alternatives, leading to the rise of synthetic data.
The Synthetic Data Solution: Feeding AI Models Their Own Output
Synthetic data is like AI teaching itself. Instead of relying solely on human-generated content, AI models create their own datasets by generating text, images, or even video. Companies like Meta and Microsoft are already using this approach to refine their AI systems.
Synthetic data has clear advantages. First, it’s scalable—AI can produce vast amounts of data in a fraction of the time it would take humans. Second, it sidesteps privacy and copyright concerns, as no human creators are involved. Third, it’s cost-effective; creating synthetic data requires fewer resources than curating and cleaning human data.
However, synthetic data comes with significant risks. One of the biggest concerns is the potential for a “feedback loop.” Picture this: if an AI model produces slightly flawed data and then trains itself on that data, those flaws get amplified over time. This could lead to a gradual degradation in the model’s accuracy and usefulness.
Risks of AI Models Feeding on Their Own Output
The most glaring risk of synthetic data is the phenomenon of “AI hallucinations,” where models generate responses that are factually incorrect or nonsensical. For instance, I once asked an AI about the history of the internet, and it confidently told me that the first email was sent in the 1800s! While it was amusing, it’s also a reminder of how unreliable AI can be when its knowledge base is compromised.
This issue is particularly concerning in fields like healthcare and law, where even minor inaccuracies can have severe consequences. Imagine an AI providing incorrect medical advice or misinterpreting legal documents—these errors could have life-altering implications.
Another concern is the loss of innovation. AI models thrive on the richness and diversity of human thought. By feeding them synthetic data, we risk creating a self-referential system that lacks the creativity and unpredictability of human input. It’s like a musician who only ever listens to their own songs—they may lose touch with the broader musical landscape, leading to repetitive and uninspired work.
Ethical and Legal Implications of Synthetic Data
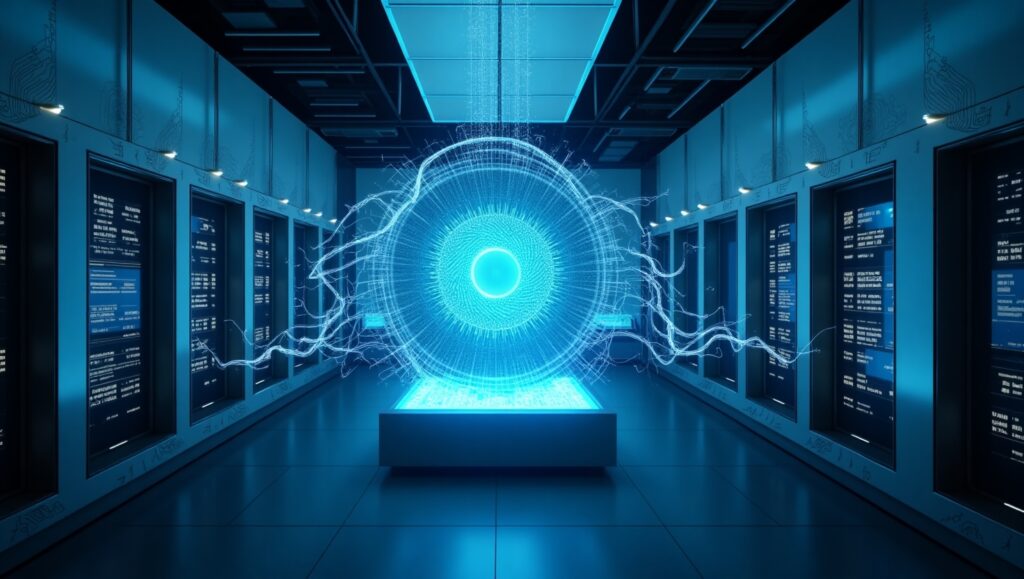
The shift to synthetic data raises a host of ethical and legal questions. One major concern is accountability. If an AI model trained on synthetic data produces harmful or misleading content, who’s responsible? Is it the developers, the data, or the AI itself?
Moreover, synthetic data can exacerbate existing biases. AI models trained on biased human data are already prone to replicating and amplifying those biases. With synthetic data, these biases could become even more deeply embedded. For example, if an AI model generates biased content and then retrains itself on that content, it creates a cycle of reinforcement that’s difficult to break.
On a personal note, I’ve seen how AI can unintentionally perpetuate stereotypes in writing. It’s subtle, but it’s there. This is why transparency in AI development is crucial. Companies must ensure that synthetic data is diverse, balanced, and free from harmful biases.
The Future of AI Models Without Human Data
The transition to synthetic data marks a pivotal moment in AI’s evolution. While it offers a way to overcome data scarcity, it also highlights the limitations of current AI models. The future of AI depends on finding a balance between synthetic and human data.
One promising approach is hybrid training, which combines synthetic data with smaller, high-quality human datasets. This method could help maintain the richness and diversity of human knowledge while addressing the scalability issues of traditional data collection.
In addition, researchers are exploring ways to make AI models more transparent and interpretable, ensuring that their outputs are trustworthy and explainable. This will be critical as AI becomes more deeply integrated into our lives.
What This Means for Society and Businesses
The shift from human to synthetic data will have far-reaching implications. For businesses, it could mean lower costs and faster development cycles. But it also requires a rethinking of strategies to ensure that AI remains reliable and ethical.
For society, the rise of synthetic data raises questions about the authenticity and value of AI-generated content. How do we ensure that AI enhances human creativity rather than replacing it? How do we maintain trust in systems that increasingly rely on self-generated data?
These are questions we must grapple with as we navigate this new chapter in AI development.
Conclusion
The exhaustion of human knowledge is a wake-up call for the AI industry. As AI models begin feeding on their own outputs, we face a unique set of challenges and opportunities. While synthetic data offers scalability and efficiency, it also introduces risks that could undermine the reliability and innovation of AI systems.
As someone who regularly interacts with AI, I’m both excited and cautious about this transition. The key to a successful future lies in finding a balance between synthetic and human data, ensuring that AI continues to serve humanity while respecting its ethical boundaries.
Ultimately, this shift is a reminder of the intricate relationship between human creativity and machine intelligence—a relationship that will shape the future of AI for generations to come.